A Fluid Flow‐Based Deep Learning (FFDL) Architecture for Subsurface Flow Systems with Application to Geologic CO2 Storage
Published in Water Resources Research, 2025
Paper: [DOI] [ResearchGate] [Download]
Code: Coming soon
Graphic Abstract
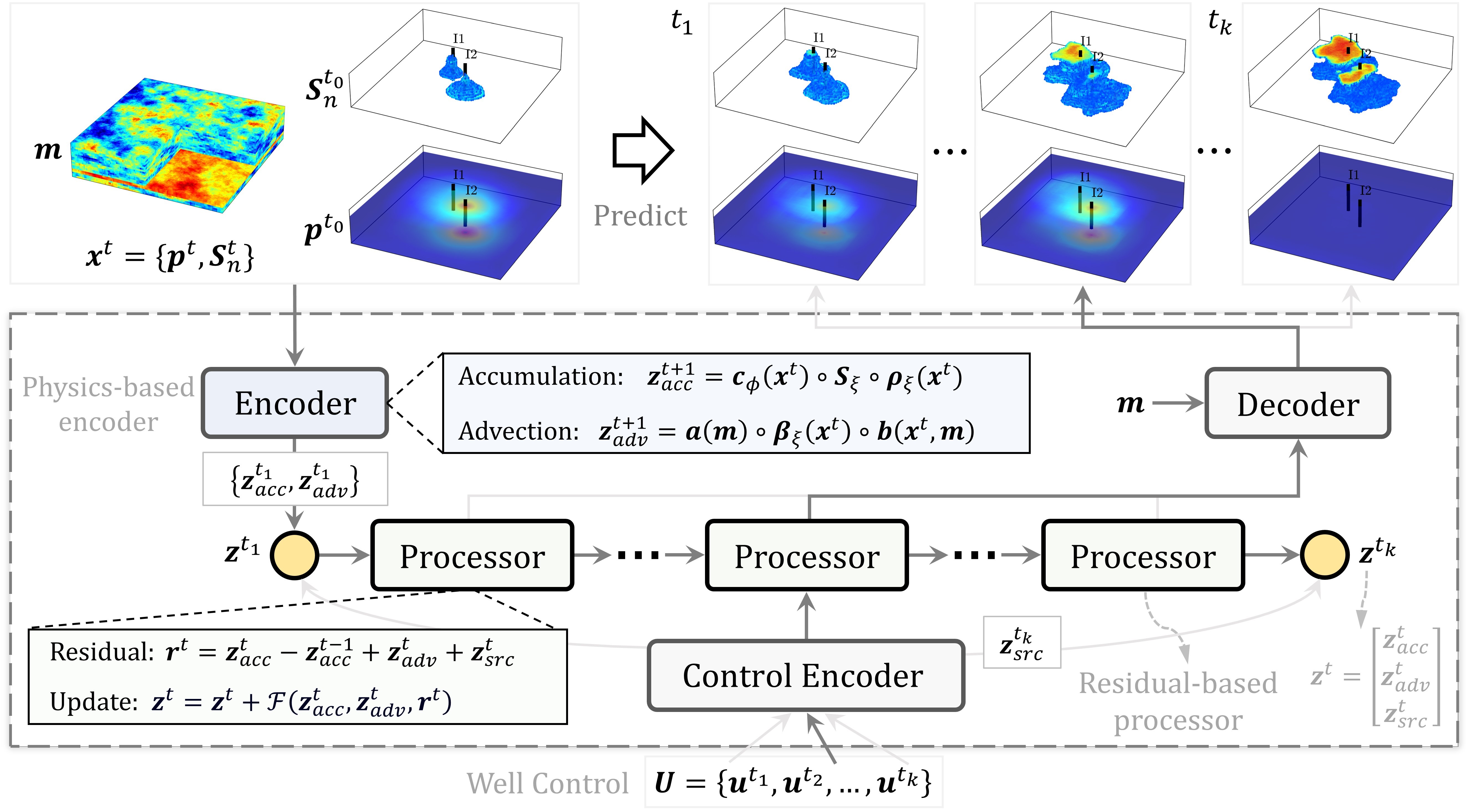
Plain Language Summary
This paper introduces a new deep learning model called Fluid Flow-based Deep Learning (FFDL) for predicting how injected CO2 moves and affects pressure within geological storage formations in carbon capture and storage (CCS) projects. Traditional numerical simulations can accurately model these processes but are computationally intensive, making real-time analysis and decision-making difficult. Existing deep learning models offer faster predictions but may lack interpretability and physical consistency. FFDL addresses these limitations by incorporating physical causality into its architecture through a physics-based encoder and a residual-based processor. This design aims to improve the model's accuracy and ensure that its predictions are more aligned with expected fluid flow behavior. Testing on a field-scale saline aquifer shows that FFDL outperforms standard deep learning models, indicating that it could be a reliable and efficient tool for decision support, optimization, and inverse modeling in CCS operations.
How to Cite
@article{qin_fluid_2025, title = {A {Fluid} {Flow}-{Based} {Deep} {Learning} ({FFDL}) {Architecture} for {Subsurface} {Flow} {Systems} {With} {Application} to {Geologic} {CO}$_2$ {Storage}}, volume = {61}, issn = {0043-1397, 1944-7973}, doi = {10.1029/2024WR037953}, number = {1}, journal = {Water Resources Research}, author = {Qin, Zhen and Liu, Yingxiang and Zheng, Fangning and Jafarpour, Behnam}, month = jan, year = {2025}, pages = {e2024WR037953}, }